Estimating gender inequalities in labor-market outcomes using mobile phone data
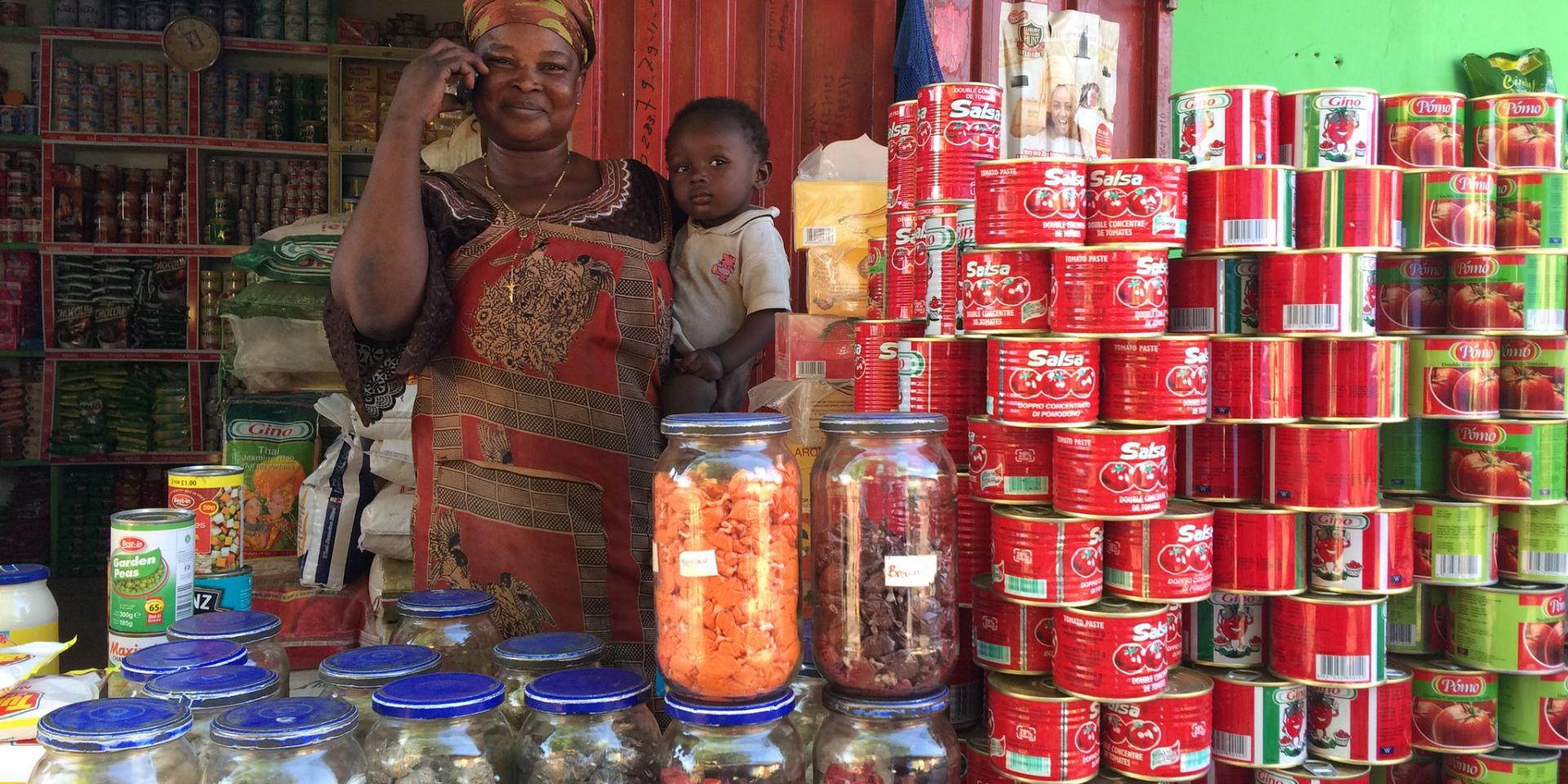
Mobile phone data holds promise for contributing to slow-filling gaps about women and men’s labor. We generated gender-specific predictions of three labor market indicators (employment, unemployment and underemployment) using machine learning models that analyzed digital trace data and geospatial data. While the models correctly predict mobile phone users’ gender in most cases, they predict users’ labor market status much less accurately. With further refinement, we believe the methodology still shows prospects for filling gender data gaps in individual-level labor market statistics.
High-quality, individual-level data on women’s and men’s labor is imperative for tracking progress on gender equality and women’s empowerment, and for evaluating development interventions that aim to improve these outcomes. Yet, using data from household surveys can be slow to fill in data gaps, especially in low- and middle-income countries. There is thus a growing need to identify new data sources and methodologies to fill gender data gaps. Big data based on digital technologies, such as mobile phones, are an emerging source with significant potential.
Mobile phones are used by 5.4 billion people worldwide and are increasingly ubiquitous in low- and middle-income countries. Digital trace data, including call detail records, are produced every time mobile phones are used—for instance, to make calls, send text messages, browse the internet, or pay using mobile money. Linked with passively collected metadata (e.g., time stamps, cell tower location), the trace data provide information on a variety of individual behaviors—including calling patterns, physical mobility and social networks—often at greater spatial and temporal resolution, and more cost-effectively, than household surveys.
In a new study, we examine the extent to which privately available, anonymized digital trace data from mobile phone networks can be used to predict labor indicators for women and men, when combined with publicly available geospatial data. Our approach differs from past work that used historical mobile phone use to predict individual characteristics/behaviors independently of the gender of the user, and vice versa (e.g., in 2022 and 2017 studies). In contrast, we jointly predict the gender and labor outcomes for mobile phone users. This is an essential extension of the methodology for gender research, because the actual user of a mobile phone may not be the same person as the registered user.
Data
We use an anonymized database of millions of mobile phone transactions from MTN Ghana (the largest mobile phone provider in the country) supplemented with a phone survey for a random sample of 8,066 MTN users plus geospatial data obtained from public databases (see table 1 for a summary). The phone survey was conducted in February 2022 in collaboration with the Ghana Statistical Service. Upon contacting users, we obtained informed consent to merge their survey responses with their mobile phone transaction history for three months before and after the phone survey. We used the phone survey data to construct individual-level indicators of employment, unemployment or underemployment.
Table 1. Summary of digital trace and geospatial data used in the analysis
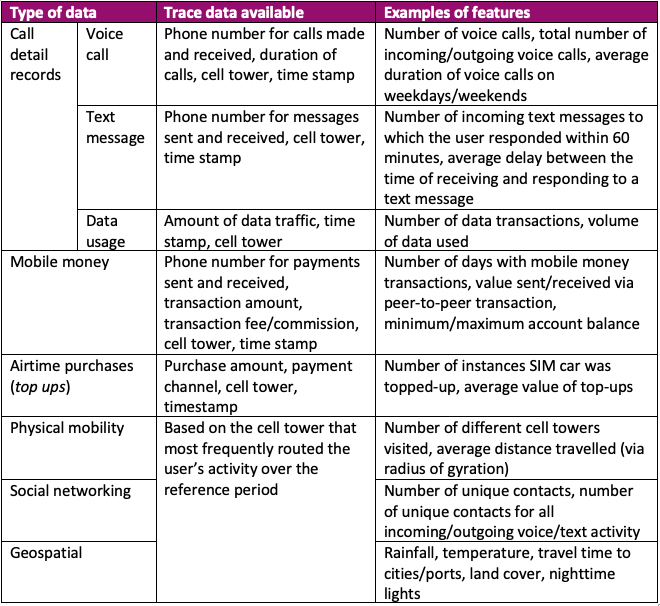
Methods
We trained machine learning models to jointly predict mobile phone users’ gender, and employment, unemployment or underemployment status based on their historical mobile phone usage. For each outcome, we generated predictions using three models: logistic regression, Bayesian additive regression trees (BART), and shared forest. The BART and shared forest are types of supervised machine learning models that differ in how they address the problem of jointly predicting users’ gender and employment, unemployment or underemployment status. The shared forest model allows for correlation between gender and labor—unlike the nonparametric BART model. Logistic regression is used as a benchmark for comparison with the machine learning models.
Results
We find that all three models predict mobile phone users’ gender at roughly the same level, in line with past studies. All three models can be expected to correctly classify mobile phone users as women in 70–71% of cases (table 2).
As an overall performance metric, area-under-the-curve (AUC)—a measure of the model’s overall ability to correctly distinguish between positive and negative results—ranges from 0.722 to 0.728 across models and outcomes.
Table 2. Cross-validation statistics showing each model’s ability to predict mobile phone users’ gender

Note: Based on a randomly selected 30% subset of data averaged across 30 replications. LR = logistic regression; BART = Bayesian additive regression tree; SF = shared forest; AUC = area-under-the-curve
Regarding the models’ ability to predict labor market outcomes, we observe a steep decline in performance (figure 1). The models can be expected to correctly classify women mobile phone users as employed, underemployed or unemployed in 52–59% of cases for women, and 52–58% of cases for men—with the AUC ranging from 0.54 to 0.63 across models and outcomes.
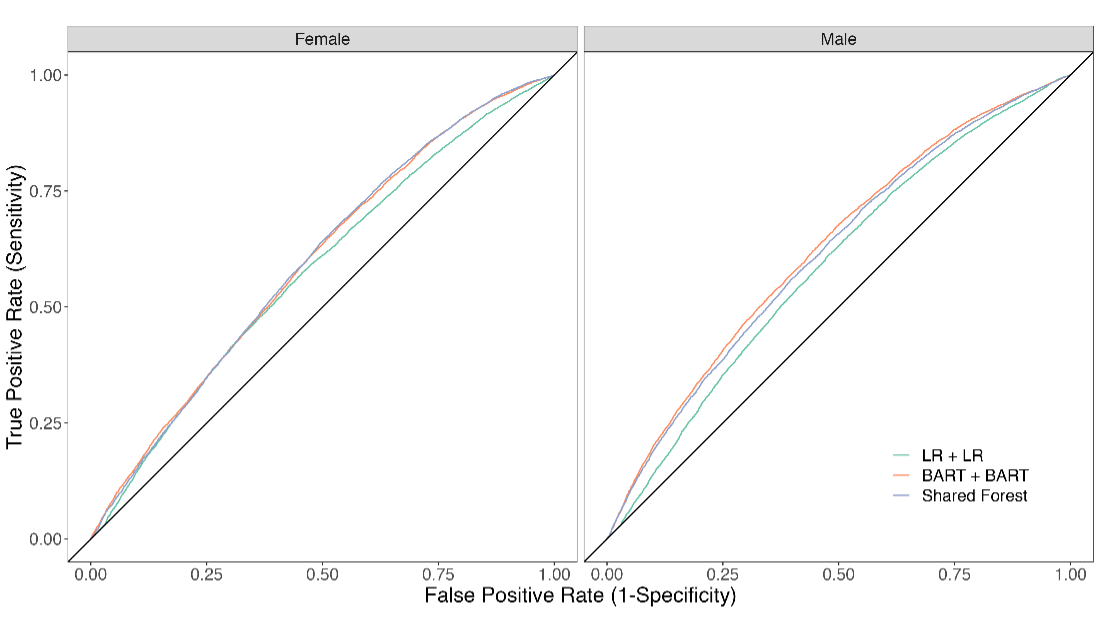
A. Employed
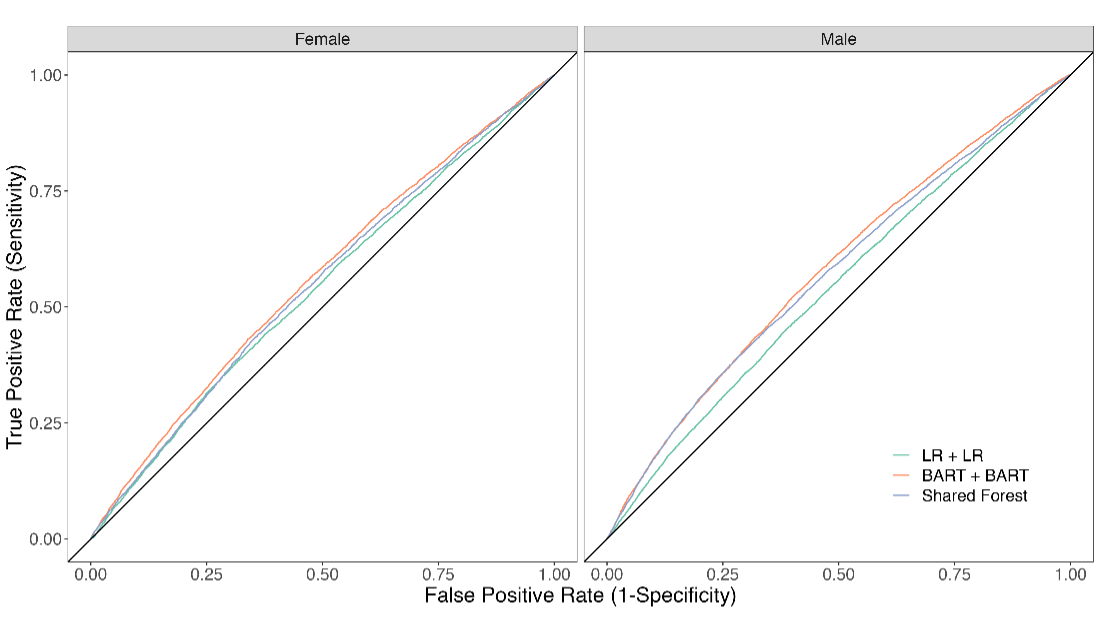
B. Underemployed
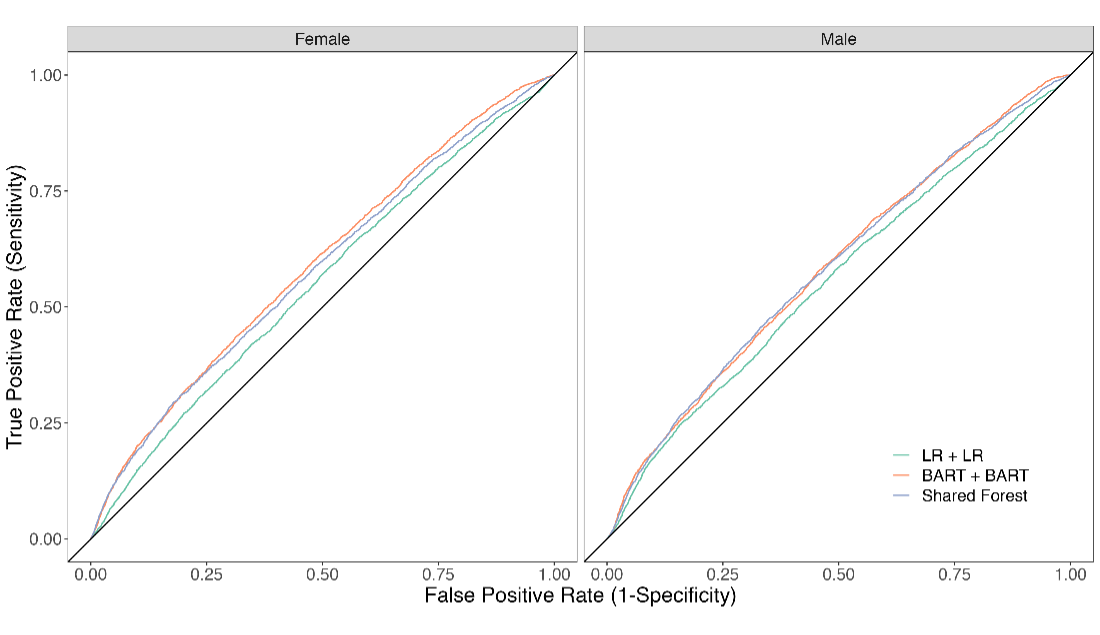
C. Unemployed
Figure 1. Receiver operator characteristic curves showing each model’s ability to predict women and men mobile phone users’ employment, underemployment and unemployment status
Since ours is the first study to apply this methodology to labor market outcomes, it is difficult to say whether this level of performance is reasonable. Our models predict labor market outcomes only slightly better than random guessing (equivalent to an AUC of 0.5), which is worse than reported by other studies in this literature, most of which aim to predict poverty status. Blumenstock et al. 2015, for instance, report an AUC ranging from 0.72 to 0.81 when predicting the poverty status of mobile phone users in Rwanda.
Our study, however, is much different. In part, this is due to the joint prediction problem we face in predicting both gender and labor outcomes. In addition, our data may be noisier. Labor outcomes are inherently more prone to short-term fluctuations than poverty status. In addition, the reference period for both the labor and digital trace data used in our study overlaps with a particularly tumultuous phase of the COVID-19 pandemic in Ghana. Both datasets, thus, almost certainly reflect disruptions in people’s behavior caused by varying COVID-19 restrictions at the time.
Despite the mixed results so far, we believe the methodology still holds promise for filling gender data gaps in individual-level labor market statistics. Looking forward, we are currently working to generate out-of-sample, gender-disaggregated predictions using the BART model for the unemployment status of the remaining 19.5 million MTN Ghana users who did not participate in the phone survey. Although publicly available individual-level data on unemployment in Ghana are only representative at the regional level, we can predict the unemployment of women and men in each of 4,256 microregions (approximating mobile phone coverage zones, generated based on cell tower locations). In addition, we are exploring options for refining the methodology and validating our predictions using data from other surveys.
##
Acknowledgements
This work was supported by the CGIAR GENDER Impact Platform and Digital Innovation Initiative. The CGIAR GENDER Impact Platform is grateful for the support of CGIAR Trust Fund contributors: www.cgiar.org/funders.